Fitch Ratings: Artificial intelligence helps underwriting but comes with risks
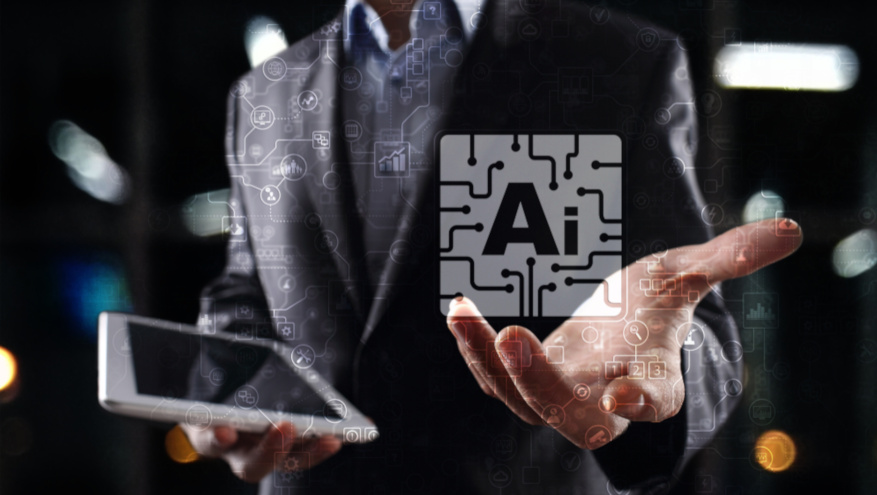
Fitch Ratings acknowledged that artificial intelligence and machine learning are increasingly deployed in credit and risk functions at financial institutions, enabled by greater data availability and affordable computing capacity
While AI and ML can improve operational efficiency and analytical outcomes, Fitch pointed out that using those tools carries risks of “black box” decision-making and data, as well as programming deficiencies and biases
Fitch elaborated about the potential risk through a report titled, “What Investors Want to Know: Artificial Intelligence and Financial Institutions,” and added that regulation and internal governance can help reduce these risks.
“Financial institutions use AI-based systems and ML to improve predictive models in operational risk management, including fraud detection, stress testing and provisioning, as well as credit assessment applications, such as credit scoring for loan underwriting and monitoring the performance of existing assets,” Fitch said in a news release.
“Regulatory reforms are being undertaken to address AI reliability and transparency issues. Underwriting criteria produced by AI models may be opaque, making it difficult to understand which factors drive the decision-making process. This also makes it difficult to compare AI model results with historical data in our analysis of structured finance transactions,” the firm continued.
Fitch also explained that the use of AI and ML can make data analysis and credit risk assessment more efficient since the tools can allows large quantities of data to be analyzed quickly and may lead to the discovery of new risk segments or patterns by filtering through variables for significant predictors.
Firm experts also said that AI and ML can expand credit availability for consumer whom creditworthiness can be measured using nontraditional metrics.
“Smaller lenders are more likely to use AI to make credit decisions, perhaps to gain an edge over competitors, and are helped by access to cloud-based lending management systems,” Fitch said.
“The quality and volume of data used to train AI/ML systems directly affects the predictive accuracy of most AI models,” Fitch went on to say. “Faulty or limited data and programmer biases can lead to erroneous AI/ML outcomes, resulting in poor origination quality, loosening underwriting practices or discriminatory credit decisions, with potential reputational and financial repercussions.”
The entire report can be found via this website.